TL;DR: Traditional Large Language Model (LLM) serving systems rely on first-come-first-serve (FCFS) scheduling. When longer requests block shorter ones in the queue, this creates a cascade of delays that severely impacts overall system latency. LLM inference jobs are particularly challenging to schedule due to their highly unpredictable workload and variable output lengths. We developed a novel learning to rank approach that predicts the relative ranking of output lengths, enabling a more efficient Shortest Job First-like scheduling policy. This scheduling approach reduced chatbot latency by 6.9x in high-load scenarios compared to commonly adopted FCFS scheduling.
vLLM-LTR
Efficient LLM Scheduling by Learning to Rank
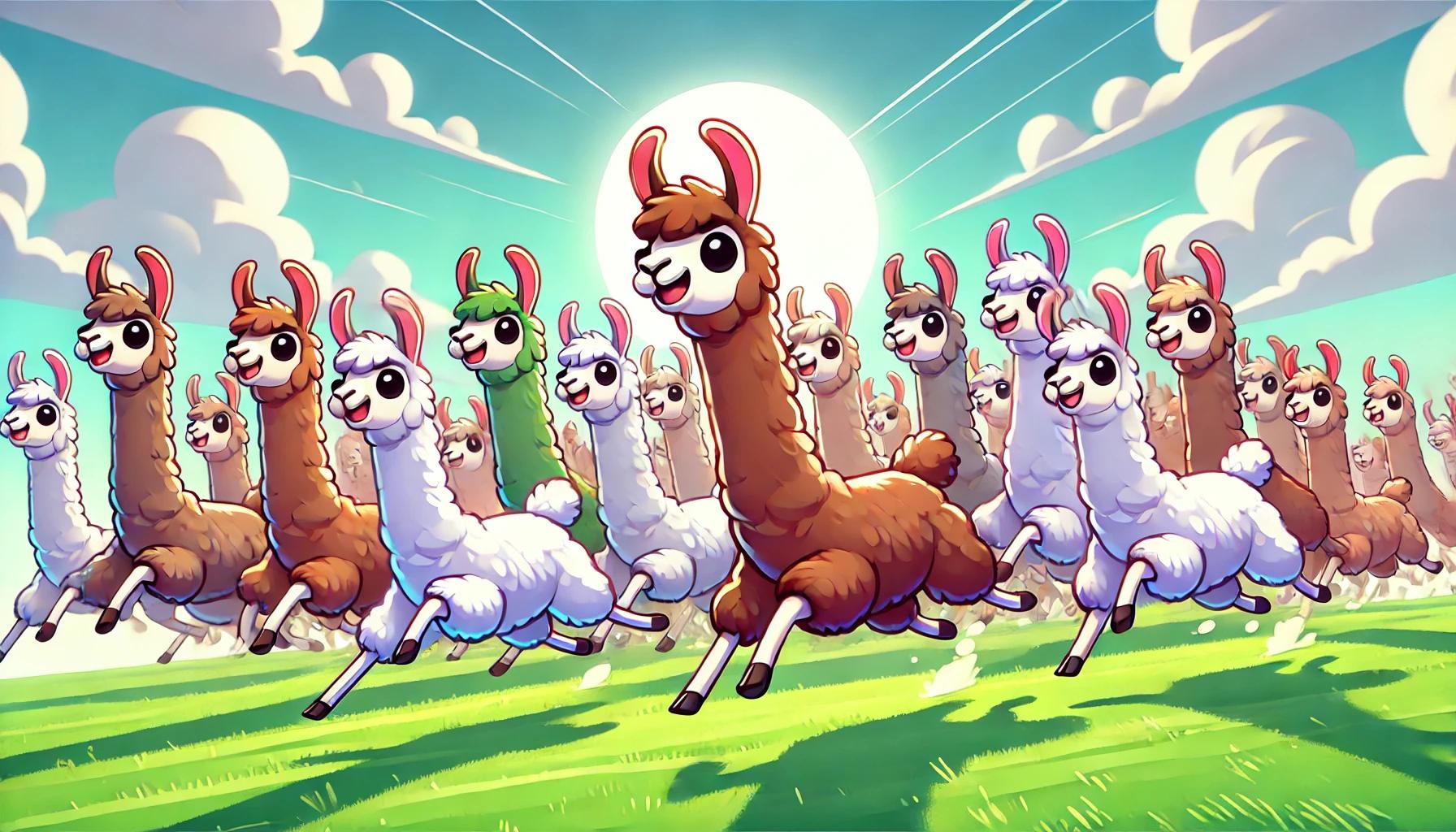